Oil and Gas
Matheron’s Mathematical Framework Applied to Uncertainty Characterization
At Seisquare, we support that the correct framework for Uncertainty Characterization is the stochastic framework described by Georges Matheron in the “Theory of Regionalized Variables” and “Estimating and choosing”. This stochastic framework enables us to translate deterministic geophysical processes into stochastic ones. The benefit is that each process feeds off uncertainty propagated from previous processes and systematically expresses and minimizes the margin of error between process outputs and true (unknown) seismic signal or subsurface properties.
The Theory of Regionalized Variables considers that the (unknown) subsurface property may be expressed as the unique outcome of a Random Function Z, defined at any location (x) in space, as a random variable Z(x):
Where Z(x) represents the true (unknown) seismic signal or subsurface property at location (x); m(x) is the average value of Z(x) at location(x); R(x)=Z(x)-m(x) i.e. the residual of Z(x) around m(x) at location(x).
Based on available scarce (well) and inaccurate (seismic) data values, it is not possible to fully determine (Z) at every location (x) in space. However, by computing experimental spatial statistics on such data sets, it is possible to determine m(x) and C(x,y) (the spatial covariance of R(x)). Characterizing m(x) and C(x,y)=Cov(R(x),R(y)) provides a mathematical ‘grip’ on (Z), that is the basis for ‘best estimating’ (Z) at any given location (x) in space. ‘Best estimating’ (Z) at any given location (x) in space is called ‘kriging’. ZK* (x0) (the kriging estimate) provides the best linear estimation of (Z) at location x0:
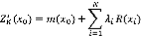
Where m(x0 ) represents the average value of ZK* (x0 ) at location (x0 ) ;

- It ensures that E{Z(x0 )- ZK* (x0) }=0 (non bias constraint). On average, the difference between the ‘kriging estimate’ and the true (unknown) seismic signal or subsurface property value is 0.
- It minimizes the estimation variance Var{Z(x0 )- ZK* (x0) }. This means that the difference between the ‘kriging estimate’ and the true (unknown) seismic signal or subsurface property is minimal.
- It ensures that reliable confidence intervals are derived from the estimation variance.
The result of kriging is a seismic signal or a subsurface property estimation that is benchmarked against its true (unknown) seismic signal or subsurface property counterpart! At any location (x), the kriging estimation expresses the most probable value for a true (unknown) seismic signal or subsurface property, and delivers the attached confidence interval.
Applying Matheron’s Stochastic Framework to Geophysical Workflows
Matheron’s stochastic framework enables us to build stochastic geophysical processes. Thes processes can be tailored to any geophysical workflow, from pre-stack processing to reservoir modeling. The beauty is that building a stochastic geophysical process always follows the same pattern:
- Step 1: elaborate specific mathematical functions for characterizing m(x) and C(x,y) (the mathematical functions must constitute a faithful translations of geophysical laws underlying geophysical workflows, such as wave propagation and compaction);
- Step 2: elaborate specific kriging (or simulation) algorithms for computing “best estimates” ZK*(x0) of the true (unknown) seismic signal or subsurface property (the kriging algorithms must constitute faithful translations of geophysical processes, such as deconvolution, filtering or time to depth conversion).
We break down these stochastic geophysical processes break down as follows:
Spatial Quality Assessment (SQA) enables us to quantify the reliability of geophysical data sets at the time of processing supervision and/or before engaging in further geophysical workflows. Usual QCs applied to geophysical data sets are about standard statistics computations. SQA offers a more robust alternative that assesses the spatial consistency of geophysical data sets by computing Spatial Quality Indexes (local spatial noise to signal ratios).
Spatial Data Conditioning (SDC) enables us to maximize the contribution of geophysical data sets before engaging in further geophysical workflows. Typical filters focus on deterministic noise removal, but the SDC process offers a more robust alternative. Based on SQA results, the SDC process provides best estimates of the spatial components from geophysical data sets corresponding to “signal”, on one hand, and to “noise” on the other.
Stochastic Geophysical Modeling (SGM) enables us to quantify and maximize the accuracy of subsurface property models. Conventional modeling methods allow the extrapolation of well data under control of geophysical data, but the SGM process offers a more robust alternative. This process provides a best estimate of subsurface property models from geophysical data under control of well data, consistently taking into account the uncertainties attached to the input geophysical data.
Conclusion
Stochastic geophysical processes ensure consistent uncertainty quantification and propagation at each and every step of geophysical workflows, and enable to convey reliable information about the subsurface. The achievement is to empower geophysicists, maximize the efficiency of E&P decision-making and contribute to sustainable and profitable Earth resource management.